Note
Click here to download the full example code
Combining COPT with JAXΒΆ
This example shows how JAX can be used within COPT to compute the gradients of the objective function.
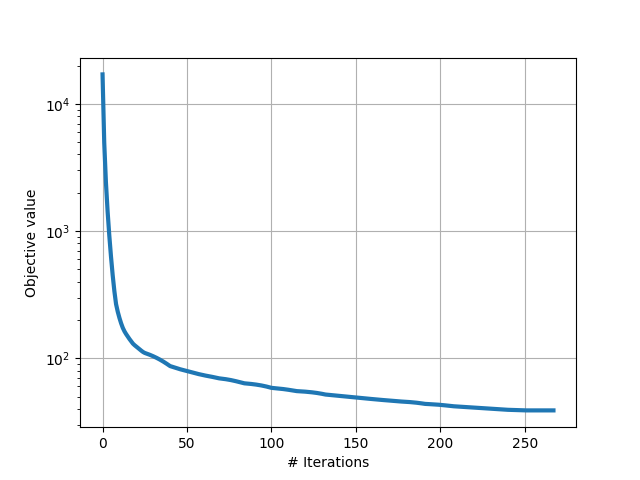
Out:
WARNING:absl:No GPU/TPU found, falling back to CPU. (Set TF_CPP_MIN_LOG_LEVEL=0 and rerun for more info.)
import jax
from jax import numpy as np
import numpy as onp
import matplotlib.pyplot as plt
from sklearn import datasets
import copt as cp
# .. construct (random) dataset ..
import copt.penalty
X, y = datasets.make_regression()
n_samples, n_features = X.shape
def loss(w):
"""Squared error loss."""
z = np.dot(X, w) - y
return np.sum(z * z) / n_samples
# .. use JAX to compute the gradient of loss value_and_grad ..
# .. returns both the gradient and the objective, which is ..
# .. the format that COPT accepts ..
f_grad = jax.value_and_grad(loss)
w0 = onp.zeros(n_features)
l1_ball = copt.penalty.L1Norm(0.1)
cb = cp.utils.Trace(lambda x: loss(x) + l1_ball(x))
sol = cp.minimize_proximal_gradient(
f_grad, w0, prox=l1_ball.prox, callback=cb, jac=True
)
plt.plot(cb.trace_fx, lw=3)
plt.yscale("log")
plt.xlabel("# Iterations")
plt.ylabel("Objective value")
plt.grid()
plt.show()
Total running time of the script: ( 0 minutes 9.940 seconds)
Estimated memory usage: 78 MB